Using OCR: How Accurate is Your Data?
With effective use of confidence scoring, you can use OCR to automate some types of data ingestion.
- By Greg Council
- March 5, 2018
All organizations want accurate data to run their operations. Getting data into a useful format is the focus of significant industry attention, whether that data comes from social media, structured databases, or unstructured documents.
Leveraging Your Document Data
One popular technology used to process documents (the scanned variety) is optical character recognition (OCR). It has been around for decades, and its most common use is to convert an image into searchable text. Obviously, the accuracy of the conversion is important, and most OCR software provides 98 to 99 percent accuracy, measured at the page level. This means that in a page of 1,000 characters, 980 to 990 characters will be accurate. In most cases, this level of accuracy is acceptable.
What about putting data from documents to good use by extracting specific data and tagging it so it can be added to a database or be used as metadata describing a specific document? Operations such as accounting rely upon accurate data from invoices (such as the invoice number, date, quantities of items purchased, and taxes).
Does the 98 to 99 percent accuracy of full-page OCR translate to an adequate level of accuracy on data extraction from these documents? Absolutely not.
Accuracy Guarantees: What It Means
If you need to obtain 99 percent accuracy at a data field level, then relying on 99 percent page-level accuracy could lead to disaster. For instance, in the case of our 1,000-character page, although an OCR engine might have 99 percent accuracy at the page level, what if those 10 erroneous characters are within 10 of the 20 data fields required by the business?
Suddenly, this 99 percent accuracy drops to 50 percent accuracy. This is where field-level accuracy comes into play, using what's known as the field-level confidence score.
Also keep in mind that page-level accuracy rates are often based upon good-quality scans. If your organization has to deal with faxed documents or documents that have hard-to-read fonts such as from a dot-matrix printer, page-level accuracy is much lower.
Why Confidence Scores Matter
In using a field-level confidence score, the main objective is to identify a "threshold" that separates good data from bad data. Good data is a "correct answer," meaning an accurate, literal transcription of the field as represented on the page. If the input document has a date of birth as 1/1/1970, the field into which the data is transcribed should contain 1/1/1970 as well.
A confidence score is assigned and output by the OCR engine for each field answer. The field-level confidence score uses the "raw" OCR character- and word-level scores and synthesizes them with other available information to arrive at a final score. This other information can be, for example, the expected data type (numerals, letters) and format (phone number versus credit card number).
For instance, the OCR engine might output the "date of birth" value as 12/5/2008 along with a confidence score of 60. The field confidence scoring for each data element should output a consistent range of scores for correct answers; these scores should be higher than the scores for incorrect answers. Although confidence scores are used to distinguish likely correct answers from likely incorrect answers, confidence scores are not probabilistic -- a score of 60 does not mean that there is a 60 percent likelihood that the answer is correct.
In reality, no OCR engine can produce a perfect correlation between a confidence score and whether or not the answer is correct. There will be instances where a correct answer has a low confidence score. Regardless, with tuned systems, the results should indicate an obvious score threshold where the majority of answers above it are correct and the majority of answers below it are incorrect.
Measure and Tune Your Data
Once we understand field-level confidence scores, we can measure and tune field-level accuracy for higher-quality data results. To be effective and reliable, this confidence score analysis should be based on several hundred to several thousand samples, ensuring the analysis includes the broadest array of variances in image quality and layout.
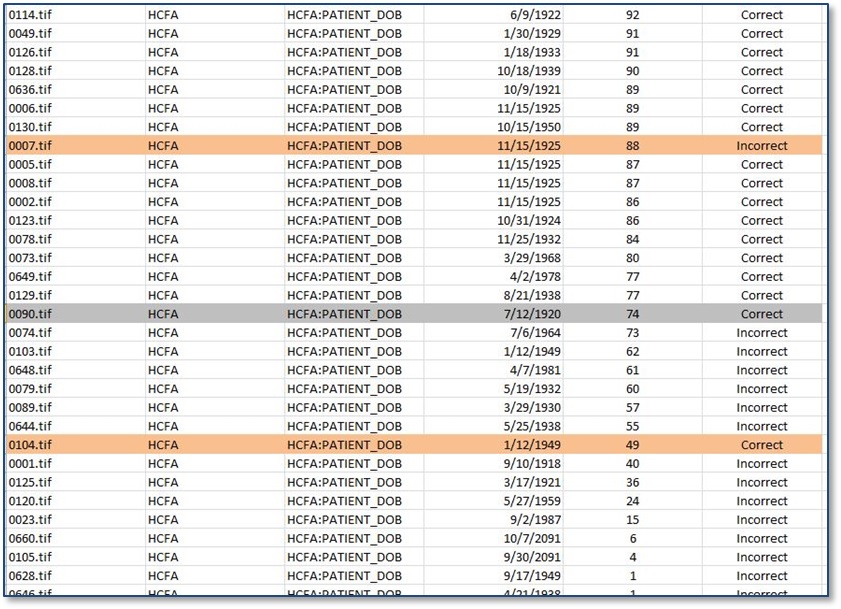
The sample table includes the image file name, the transcription of the field (date of birth) from the OCR engine, and the confidence score for that field (also generated by the OCR engine). The final column shows the judgment from an analyst as to whether the OCR answer matches exactly what is on the document image.
Identifying the threshold requires this step -- human analysis of the OCR output that evaluates where the OCR engine was accurate versus inaccurate. Once this is done, answers can be ordered by confidence score from high to low in order to identify the optimal threshold. To find the optimal threshold, you must calculate accuracy provided at a specified threshold. We measure actual accuracy of a specified threshold by dividing the number of OCR answers above the threshold that are accurate by all answers provided above the threshold.
In this scenario, all but one answer with a score equal to or above 74 are correct. There is also an answer below the threshold of 74 that is correct. Therefore, the majority of data can be segmented into two groups: one with a field-level confidence score of 74 or above and one group with scores of less than 74. Separation of data into these two groups is the goal of using confidence scores.
This ability for OCR to consistently output reliable confidence scores (i.e., erroneous data consistently has lower confidence scores than accurate data) to determine breakpoints is called establishing confidence thresholds and allows for true automation of data extraction, ensuring high accuracy and completely removing the need for manual verification of the majority of your data. Only data that falls below the identified confidence threshold (74 in this case) is probably inaccurate and must be manually reviewed.
Note that due to differences in data fields, it is entirely possible and realistic that some fields can use a low confidence threshold while others require a higher threshold; it all depends upon the analysis. Perhaps "date of birth" can have a threshold of 74, but the Social Security field needs a threshold of 88.
Consistency with Scores
In some cases, OCR software cannot produce sufficiently consistent field confidence scores to establish an ordered list of answers that allow selection of a single confidence score threshold (where answers above the threshold are mostly accurate). When confidence scores are unreliable, an ordered list of answers based upon confidence scores will produce many incorrect answers above and correct answers below any threshold.
When this is the case, rather than having accurate data to flow through from OCR to, say, the data warehouse without the need for verification, all data is forced to go through manual review. Even if most of the data is correct, the extra review time is costly, and there is a higher probability that manual review will not identify all incorrect data due to human error.
Moving Beyond Generic OCR
Application of field-level OCR requires a deeper level of analysis and tuning that may not always be available in off-the-shelf OCR software, so it is important to know if the software you are evaluating can support field-level tuning based on reliable confidence scores. Most vendors will provide evaluation software so you can test this capability.
To truly achieve automation with minimal manual review requires attention to field-level confidence scores and the ability to identify a threshold that reliably separates good data from bad. Although generic page-level OCRs are useful to meet certain business needs, they are limited in field-level accuracy, so it is important to evaluate field-level answers along with confidence scores to identify if the output can produce "reliable enough" confidence scores to establish an optimal confidence score threshold.
There is also a way to boost the OCR-generated score to increase reliability as well as automate identification of the appropriate thresholds, which we will discuss in Part 2. The benefit is a significant reduction in manual labor costs.