The Five Ds for AI Project Deployment Success
Adopting AI-enabled technologies requires more than buying a license. Enterprise IT groups need to examine their readiness for AI-led innovation using the five Ds of AI deployment.
- By Stan Pugsley
- September 13, 2023
The artificial intelligence (AI) rush is on and is headed for the enterprise. As AI-driven excitement and hype spreads through financial markets and investors, every imaginable software company will jump into the arena with real and sometimes vapor-ware enhancements to their products. The marketing efforts from these software vendors will increase as each vies for attention and corporate budgets. Next, pressure will increase on information executives (CIOs, CTOs, CISOs, and similar roles) to find and deploy the technologies to improve competitiveness and reduce costs. In the rush to embrace the new technologies, you must understand how to evaluate your organization’s readiness for AI automation and target the business areas most likely to succeed.
To function correctly, AI deployments depend on five requirements, described in Figure 1.
Let’s look more closely at each D on the list.
Defined Process
Before engaging in any project, business stakeholders must agree on how the process works and how it should ideally work. What is the desirable outcome? How should exceptions be handled?
Automation of any process depends on understanding the process. To illustrate, let’s say your organization has an informal planning and budgeting process that varies depending on the mood of the owner. In this business function, you will struggle to find a way to identify any improvements using AI. On the other hand, if you have a well-structured planning process with consistent variables and clear KPIs, you will have much greater success automating the process.
Digitized Tasks
Manual or offline tasks will be the high-hanging fruit for AI. It could take months of effort to understand them, design applications to support them, and monitor the outcomes. A task that has been migrated to an application or database will be much more easily understood and automated in an AI platform.
Data Collected
AI-driven processes have data as their foundation. Prior to automating a process, you should have at least three months’ worth of data collected for non-cyclical processes and one to two years for cyclical processes. This data will guide the design, implementation, and control of any process. Data collected in an unstructured data lake is a good start, but a conformed, integrated data set is essential to understanding all inputs and outputs for a process.
A data warehouse will accomplish the task of conforming and integration but will lack the overall semantic model that gives business context. The ideal platform for AI-automation is a knowledge graph, with interconnected processes in documented relationships.
Deployment Path
Evaluate how the AI software will be deployed. For off-the-shelf solutions, would the AI app be compatible with existing technologies in your organization or would it require extensive integration and consulting work? For custom-built solutions using open-source tools, do you have the resources and skills to successfully implement and sustain a solution? Organizations that already host data and applications in the cloud will have an advantage in integrating new AI-driven software, which is most often native to the cloud.
Demand
The final requirement is business value for and interest in (or demand for) the project. Is this project significant to revenue or expenses in the organization? Will it move the needle enough to justify the effort and expense in the deployment? Early in its AI journey, your organization may have set a low bar for proving ROI of innovation projects, but that blank check will quickly end if results are not proven.
The Five Ds in Practice
To illustrate these requirements, let’s use as a case study of a consumer products company that seeks to generate hyper-customized marketing content for each customer based on the behavioral, transactional, and demographic information for the customer.
Using a generative AI tool such as ChatGPT or Bard, the enterprise could connect to the API, provide information about the customer, and clearly define the format of the content. The enterprise could also request an optimized marketing plan for the customer. The output from the AI tool could be used to help could generate targeted email, social media, or paid marketing content for the customer, and designate the micro-campaign variables. The goal would be to increase the clickthrough rates and purchases from the customer.
Using our 5 Ds, we can evaluate whether the content marketing processes are ready and likely to be successful in an AI deployment. We may decide that the high-level budgeting project for overall marketing costs it too ill-defined to be automated. However, down a layer -- at the campaign planning and execution level -- the tasks have been digitized in various software packages, several years of well-organized historical data are available, the target outcome is known (higher sales and lower ad spending), and your organization can integrate with the ChatGPT API with a Python connection and campaign workflow automation tool. The final step is to look at reference projects or conduct a small pilot project to determine how much time can be saved in campaign management and how much of a lift in customer spending could be expected. If all these elements are in place, the project has a high likelihood of success.
Finally, it is important to consider that the AI deployment stack consists of four layers, with data as the foundation. Fortunately, the modeling/algorithm layer will be funded by Wall Street investors and academic research. The application layer will quickly be filled by software vendors extending their current products or introducing new tools with API connections. However, there is no way to buy or outsource the data or business processes. The internal capabilities in designing business processes and utilizing data will determine which organizations will be the winners and losers in the AI-driven innovation race.
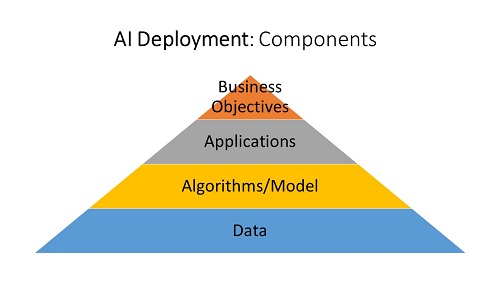
As you examine the various functions in your organization for potential candidates for AI automation, you can use the 5 Ds to score the readiness of each business process and focus on those areas most likely to be successful. The best preparation for future automation projects is to start today and digitize your offline and manual processes and invest in collecting, integrating, and managing your data.
About the Author
Stan Pugsley is an independent data warehouse and analytics consultant based in Salt Lake City, UT. He is also an Assistant Professor of Information Systems at the University of Utah Eccles School of Business. You can reach the author via email.